Dynamic recalibration of a disease spread model
Modelling of disease spread was a key component of protection of public health and planning during the COVID-19 pandemic. Reduction in the inherent uncertainty in such model predictions is a priority, and this can be achieved by incorporating the most recent available real-world data into the predictions. This study used an Approximate Bayesian Computation (ABC) method to dynamically recalibrate a disease spread model based on emerging new data during the pandemic, and showed that forecasts of future disease infection rates are improved substantially. The work was undertaken using the Faculty of Environment foe-linux intensive compute cluster.
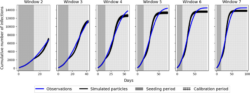
Comparing the cumulative number of infections predicted by the model (black lines; one for each particle) to those observed in the real data (blue line).
Reference
Asher, M., Lomax, N., Morrissey, K. et al. Dynamic calibration with approximate Bayesian computation for a microsimulation of disease spread. Sci Rep 13, 8637 (2023). https://doi.org/10.1038/s41598-023-35580-z